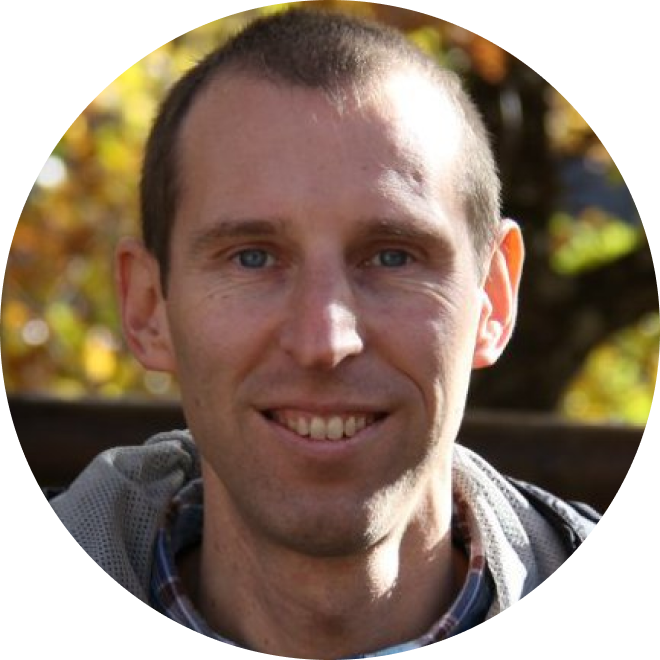
Konstantin Avrachenkov
Venue: TII Yas Auditorium
6 November 2024, 10:00 AM -11:00 AM (GST)
Title: | Graph-based Methods for Unsupervised and Semi-supervised Learning |
Abstract: | Graph-based methods are powerful tools in both unsupervised and semi-supervised learning due to their ability to model complex relationships and capture the underlying structure of data. This talk explores key techniques for applying graphs to data analysis and learning tasks. For unsupervised learning, I will discuss clustering techniques such as spectral clustering and modularity-based methods. These methods use the structure of graphs to discover meaningful patterns in unlabelled data. In the context of semi-supervised learning, I will explore algorithms like label propagation and random walk based methods, which efficiently spread information across graphs to improve learning performance when some labelled data is available but scarce. When both graph structure and some nodes' attributes are available, I recommend to use Graph Neural Networks. Throughout the talk, I will emphasize the practical applications of these methods and their relevance to real-world problems, ranging from social network analysis to electrical grids. |
Bio: | Konstantin Avrachenkov received Master degree in Control Theory from St. Petersburg State Polytechnic University (1996), Ph.D. degree in Mathematics from University of South Australia (2000) and Habilitation from University of Nice Sophia Antipolis (2010). Currently, he is a Director of Research at Inria Sophia Antipolis, France. He is an associate editor of Probability in the Engineering and Informational Sciences, Stochastic Models, ACM TOMPECS, IEEE Trans on Automatic Control, and on advisory boards of ACM POMACS and International Journal of Performance Evaluation. Konstantin has co-authored two books “Analytic Perturbation Theory and its Applications”, SIAM, 2013 and “Statistical Analysis of Networks”, Now Publishers, 2022, and more than 200 articles. He has won 5 best paper awards. His main theoretical research interests are Markov chains, Markov decision processes, random graphs and singular perturbations. He applies these methodological tools to the modelling and control of networks, and to design data mining and machine learning algorithms. |