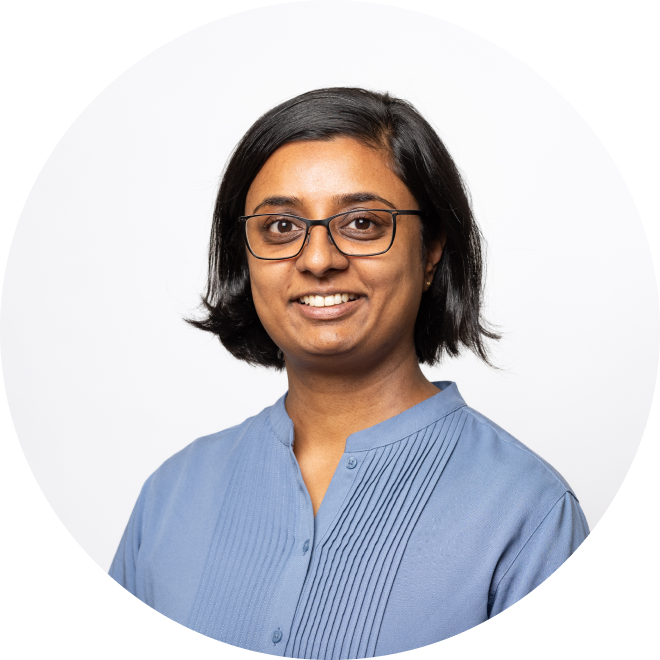
Veelasha Moonsamy
Ruhr University Bochum (Germany)
CASA, Martin Steffen
8th January 2025, 4:00PM - 5:00PM (GST)
Title: | Federated Learning and Its Application for a Privacy-Respecting Android Malware Classifier |
Abstract: | Federated Learning (FL) has gained popularity as a mechanism to address privacy threats in the training process of a machine learning model. Instead of sharing raw data, users can share locally trained models to prevent service providers from getting access to their personal information. In this talk, I will introduce the basics for understanding FL and discuss three important shortcomings of vanilla FL: Firstly, users are required to provide the system with ground-truth to enable local training on their own devices. Secondly, the introduction of malicious users to the federation may break the integrity of the model in order to lower performance. Lastly, an honest-but-curious service provider may break users' privacy by attacking their individual models. Our proposed solution is based on semi-supervised machine learning techniques that, on the one hand, allow users to learn from their unlabeled data, and on the other hand, reduce the attack surface of the federated model. We demonstrate the feasibility of our design by implementing LiM, an Android malware classifier that is resistant against poisoning and inference attacks while providing state-of-the-art results without user supervision. I will conclude by giving an overview of potential applications of LiM beyond malware detection. |
Bio: | Veelasha Moonsamy is a Professor in the Faculty of Computer Science at Ruhr University Bochum (Germany), where she leads the Chair for Security and Privacy of Ubiquitous Systems. She is also a member of the Horst Goertz Institute for IT Security and a Principal Investigator in the Excellence Cluster CASA. Her research interests include security of IoT/mobile/embedded systems, data privacy and applications of machine learning for security and privacy. |