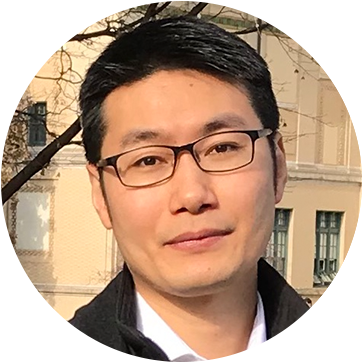
Kun Zhang
Mohamed bin Zayed University of Artificial Intelligence & Carnegie Mellon University
6th October 2022 4:00pm - 5:00pm (GST)
Title: | Methodological advances in causal representation learning |
Abstract: | Causal representations help understand the underlying causal process, facilitate downstream tasks, and provide one way to achieve explainable AI. Causal representation learning aims to reveal the underlying high-level hidden causal variables and their relations. It can be seen as a special case of causal discovery, whose goal is to recover the underlying causal structure or causal model from observational data. The modularity property of a causal system implies properties of minimal changes and independent changes of causal representations, and how such properties make it possible to recover the underlying causal representations from observational data with identifiability guarantees: under appropriate assumptions, the learned representations are consistent with the underlying causal process. The talk will consider various problem settings involving independent and identically distributed (i.i.d.) data, temporal data, or data with distribution shift as input, and demonstrate when identifiable causal representation learning can benefit from the flexibility of deep learning and when it has to impose suitable parametric assumptions on the causal process. |
Bio: | Kun Zhang is an associate professor of machine learning at Mohamed bin Zayed University of Artificial Intelligence (MBZUAI). He is on leave from Associate Professorship duties at Carnegie Mellon University (CMU). He has been actively developing methods for automated causal discovery and causal representation learning from various kinds of data and investigating machine learning problems including transfer learning, representation learning, and reinforcement learning from a causal perspective. He co-authored a best student paper for UAI 2010 and a best finalist paper for CVPR 2020, and received the best benchmark award of the 2nd causality challenge in 2008 and Test of Time Honourable Mention at ICML 2022. He has been frequently serving as a senior area chair, area chair, or senior program committee member for major conferences in machine learning or artificial intelligence, including NeurIPS, ICML, UAI, IJCAI, AISTATS, and ICLR, and is a general & program co-chair of the first Conference on Causal Learning and Reasoning (CLeaR 2022) and a program co-chair of the 38th Conference on Uncertainty in Artificial Intelligence (UAI 2022). |